This project follows up on the VDPA+ internship
This project focuses on assessing the reliability and robustness of a sunflower crop model when tested under extreme conditions, with the aim of predicting sunflower sensitivity to climate change and the evolution of its production areas.
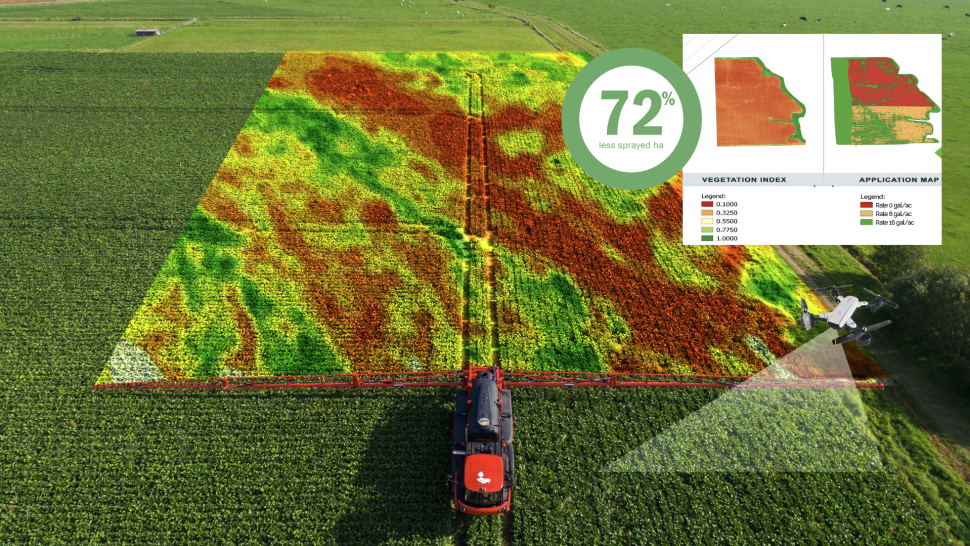
Projet background
Predictive models, algorithms, and artificial intelligence (AI) are increasingly integral to the agricultural ecosystem. These systems rely on large quantities of high-quality data to train models effectively. Additionally, environmental conditions are changing significantly, often presenting more frequent extreme events (such as floods, heatwaves, and droughts).
Given these challenges, critical questions arise:
- How do our AI systems and models perform under extreme conditions?
- How robust are these models, and how can we integrate all the necessary data to configure them effectively?
The SUNFLO model is a crop model specifically designed for sunflower cultivation. Developed by INRAe (Casadebaig, 2011), this model simulates the behavior of sunflower plants using a variety of climatic, soil, and other parameters. The configuration of this model’s parameters is based on extensive datasets obtained from field trials. However, the performance of a model outside its training zone always poses a risk to the quality of predictions.
La configuration des paramètres de ce modèle repose sur de nombreux jeux de données acquis lors d’essais. Mais le comportement d’un modèle en dehors de sa zone d’apprentissage représente toujours un risque sur la qualité des prédictions.
This project aims to explore how the SUNFLO model and similar AI-driven models behave under extreme conditions, ensuring that predictions remain reliable even when environmental factors deviate significantly from the norm. The project will also investigate methods to integrate and manage the extensive datasets required to fine-tune these models effectively, contributing to a more resilient agricultural sector in the face of climate change.
A model’s behavior outside its training zone always poses a risk to the accuracy of its predictions. The primary objective of this project is to assess how sensitive a model is to “extreme” input data, especially when such data falls outside the model’s training range.
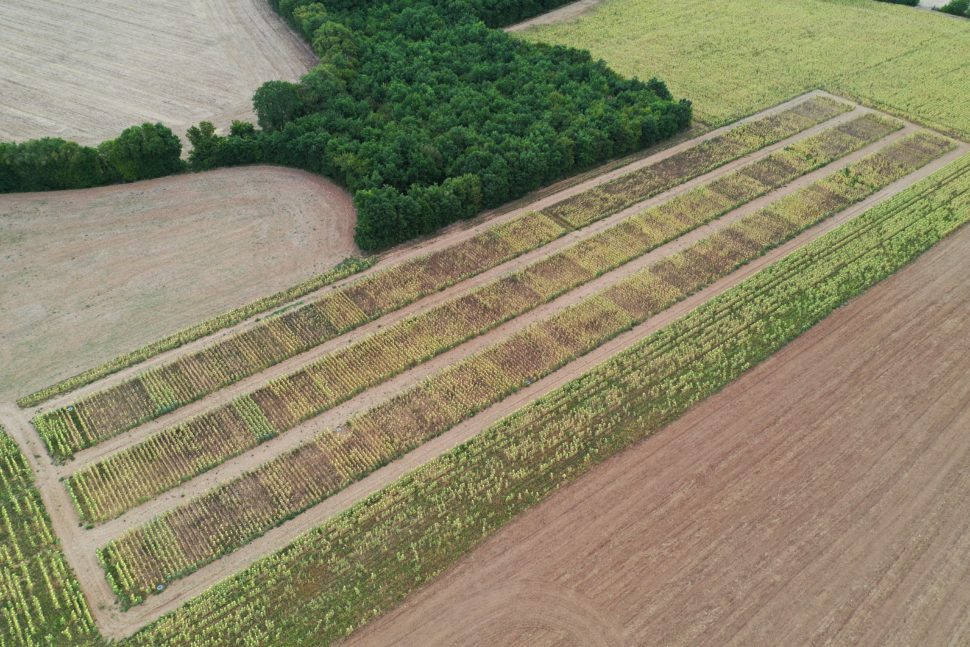
Project objectives
- The primary objective of this project is to assess how sensitive a model is to “extreme” input data, especially when such data falls outside the model’s training range.
- To achieve this, the project will apply a well-established model like SUNFLO to generate predictive results using data gathered by the partners of the Alliance H@rvest. Data integration is a crucial aspect of this project, serving to illustrate the outcomes of Dafa Yumna’s internship, which concluded at the end of 2023, with results available on the VDPA+ project page.
- One practical application of these predictions will be a yield gap study, aiming to measure the discrepancies between predicted and actual yields.
Complementary Actions to Research
- Data Integration Challenge: Implement a “delicate” data integration case, focusing on the rules for sharing and accessing non-open sources provided by the alliance and other suppliers. This includes engaging with the sources identified by Dafa Yumna.
- AFNOR Standardization Process: Participate in the AFNOR standardization process on behalf of the Alliance, providing feedback within the Alliance and to certain members. Use this “use case” as a discussion tool.
Expected project outcomes
Examples of applied valorization products include:
- A sensitivity map for sunflowers under one or more climate change scenarios, highlighting areas where sunflowers are most vulnerable to changes in climate conditions.
- The evolution of sunflower production zones, projecting how these regions may shift or expand due to climate change.